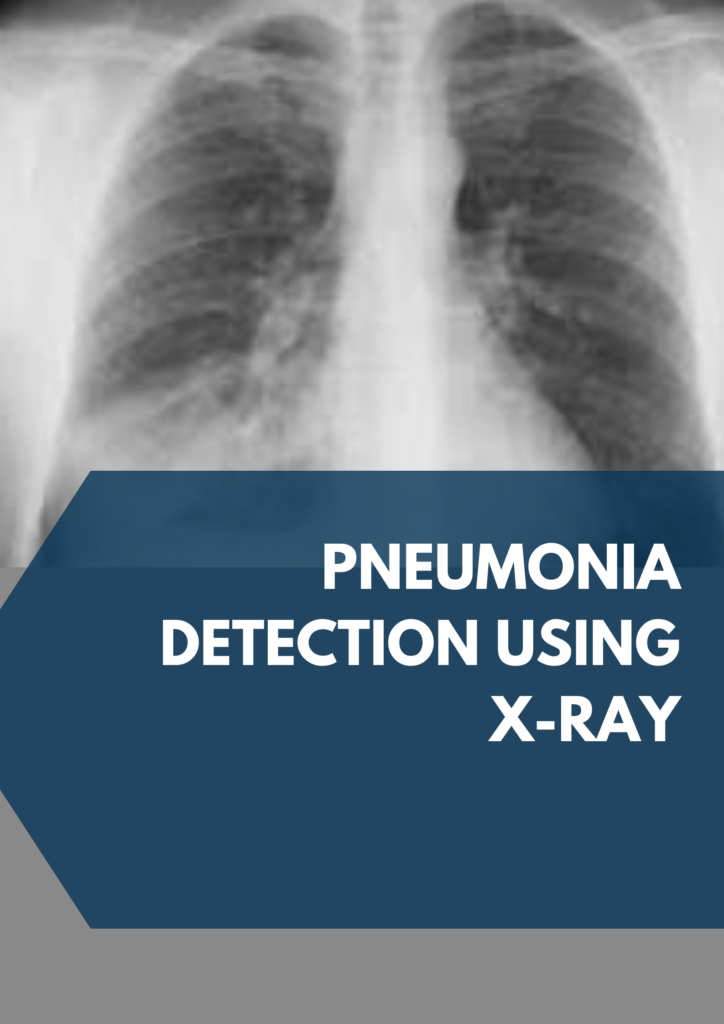
Abstract:
Pneumonia is a prevalent and potentially life-threatening respiratory infection that requires prompt and accurate diagnosis for effective treatment. Traditional methods of pneumonia diagnosis, such as visual inspection of chest X-ray (CXR) images by radiologists, are time-consuming and may be prone to human error. In this study, we propose an automated pneumonia detection system utilizing deep learning techniques to enhance the efficiency and accuracy of diagnosis.
Our approach involves the use of Convolutional Neural Networks (CNNs) to analyze and classify chest X-ray images into pneumonia-positive or pneumonia-negative categories. We employ a large dataset of annotated chest X-ray images to train and fine-tune the CNN model, allowing it to learn intricate patterns and features indicative of pneumonia.
The trained model exhibits promising performance in terms of sensitivity, specificity, and overall accuracy during validation and testing phases. We also explore the interpretability of the model by visualizing the areas of interest in the X-ray images that contribute to the classification decision. The proposed system demonstrates its potential as a valuable tool for assisting healthcare professionals in the early and accurate detection of pneumonia, thereby facilitating timely intervention and improving patient outcomes.
The integration of this automated pneumonia detection system into clinical workflows has the potential to expedite diagnosis, reduce the burden on healthcare practitioners, and enhance the overall efficiency of pneumonia management. Future work may involve further refinement of the model, validation on diverse datasets, and exploration of real-world implementation challenges to ensure its seamless integration into healthcare settings.